|
|
Tutorials
As for all previous PAKDD conferences this year
again we organised a tutorial program designed to provide attendees
an overview of the state-of-the-art and practical applications in
important current areas in Knowledge Discovery and Data Mining.
Due to space limitations we could only select three from the many
submitted high quality tutorial proposals which came all from
distinguished researchers in their respective field.
Each tutorial will run for three hours, in the morning of 20 May
2008, or in the afternoon. The topics are on Data Stream Mining,
Detecting Clusters in Moderate to High Dimensional Data, and Web
Spam Detection. These were selected in order to offer the attendees
of this year's PAKDD overviews of a broad spectrum of important
current and emerging research and application areas. We believe
attendees will be able to significantly benefit from the tutorials
on offer.
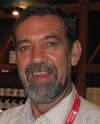 |
Prof. Joao Gama
Laboratory of Artificial Intelligence and Decision Support,
INESC-Porto, University of Porto, Portugal
Homepage:
http://www.liaad.up.pt/~jgama/ |
Title:
State-of-the-art in Data Stream Mining
Abstract:
Data streams became ubiquitous as many sources produce data
continuously and rapidly. Examples of streaming data include
sensor networks, customer click streams, telephone records, web
logs, multimedia data, sets of retail chain transactions, etc.
These data sources are characterized by continuous generating
huge amounts of data from non stationary distributions. Data
Streams have brought new challenges to the data mining research
community. In consequence, new techniques are needed to process
streaming data in reasonable time and space. The goal of this
tutorial is to present and discuss the research problems, issues
and challenges in learning from data streams. We will present
the state-of-the-art techniques in change detection, clustering,
classification, frequent patterns, and time series analysis from
data streams. Applications of mining data streams in different
domains are highlighted. Open issues and future directions will
conclude this tutorial. The tutorial also points to data stream
mining resources.
Short Biography:
Joao Gama is a researcher at LIACC, the Laboratory of Artificial
Intelligence and Computer Science of the University of Porto,
working at the Machine Learning group. His main research interest is
in Learning from Data Streams. He has published several articles in
change detection, learning decision trees from data streams,
hierarchical Clustering from streams, etc. Editor of special issues
on Data Streams in Intelligent Data Analysis, J. Universal Computer
Science, and New Generation Computing Co-chair of a series of
Workshops on Knowledge Discovery in Data Streams, ECML 2004, Pisa,
Italy, ECML 2005, Porto, Portugal, ICML 2006, Pittsburg, US, ECML
2006 Berlin, Germany, SAC2007, Korea, and the ACM Workshop on
Knowledge Discovery from Sensor Data to be held in conjunction with
ACM SIGKDD 2007. Gama and Gaber edited the book: Learning from Data
Streams-Processing Techniques in Sensor Networks, published by
Springer.
Mohamed Medhat Gaber is a research scientist at Commonwealth
Scientific and Industrial Research Organization (CSIRO), Australia.
He has published more than 40 articles. Mohamed has served in the
program committees of several international and local conferences
and workshops in the area of data mining. He has also been serving
as a reviewer for the special issues of international journals in
the area of data stream mining. He was the co-chair of the
International Workshop on Mining Evolving and Streaming Data held in
conjunction with ICDM 2006. He is the co-chair of the International
Workshop on Knowledge Discovery from Ubiquitous Data Streams to be
held in conjunction with ECML/PKDD 2007 and the ACM Workshop on
Knowledge Discovery from Sensor Data to be held in conjunction with
ACM SIGKDD 2007. Gama and Gaber edited the book: Learning from Data
Streams-Processing Techniques in Sensor Networks, published by
Springer.
|
Title:
Detecting Clusters in Moderate-to-High Dimensional Data Subspace
Clustering, Pattern-based Clustering, and Correlation Clustering
Abstract:
This tutorial provides a comprehensive and comparative overview
of a broad range of state-of-the-art algorithms for finding
clusters in moderate-to-high-dimensional data. It sketches
important applications of the introduced methods, outlines the
general challenges these algorithms have to cope with, and
presents a taxonomy of existing approaches. In addition,
relationships between the algorithmic approaches of each
category of the taxonomy are discussed. The intended audience of
this tutorial ranges from novice researchers to advanced experts
as well as practitioners from any application domain dealing
with high-dimensional data.
Short Biography:
(to be announced)Hans-Peter Kriegel is a full professor for
database systems and data mining in the Department ``Institute for
Informatics'' at the Ludwig-Maximilians-Universitaet Muenchen,
Germany and has served as the department chair or vice chair over
the last years. His research interests are in spatial and multimedia
database systems, particularly in query processing, performance
issues, similarity search, high-dimensional indexing as well as in
knowledge discovery and data mining. Kriegel received his MS and
Ph.D. in 1973 and 1976, respectively, from the University of
Karlsruhe, Germany. Hans-Peter Kriegel has been chairman and program
committee member in many international database and data mining
conferences. He has published over 200 refereed conference and
journal papers, and he received the ``SIGMOD Best Paper Award'' 1997
and the ``DASFAA Best Paper Award'' 2006 together with members of
his research team.
Peer Kroeger is an assistant professor at the Ludwig-Maximilians-Universitaet
Muenchen, Germany. He finished his PhD thesis on clustering
moderate-to-high dimensional data in summer 2004 and is currently
working towards his Habilitation with research interests in data
mining and similarity search in high dimensional multimedia and
biomedical data. Several of his recent publications include
contributions to the tutorial's scope.
Arthur Zimek is a PhD student in the database and data mining group
of Hans-Peter Kriegel at the Ludwig-Maximilians-Universitaet
Muenchen, Germany. His research interests include data mining for
high dimensional data and structured data especially for
bioinformatics applications. Several of his recent publications
include contributions to the tutorial's scope.
|
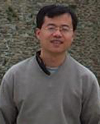 |
Dr. ZhaoHui Tang
Microsoft, U.S.A.
|
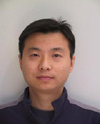 |
Mr. Dylan Hai Huang
Microsoft, U.S.A.
|
Title:
Data Mining Techniques for Web Spam Detection
Abstract:
Web spam, which refers to any deliberate actions bringing to
selected web pages an unjustifiable favorable relevance or
importance, hurts the quality of information retrieval on the web.
The tricks of web spam can be roughly divided into two categories:
content spam and link spam. Content spam tricks disguise the content
of a web page so that it appears relevant to many popular searches.
Link spam tricks boost page rankings by making up some linkage
structures. Interdisciplinary data mining techniques have been
extensively used to combat web spam. However, as web spam tricks
become more and more sophisticated, effective and efficient data
mining techniques dedicated for web spam detection are critical, and
core data miners are highly wanted. It is high time the data mining
community seriously addressed the demand of data mining techniques
for spam detection. In this tutorial, we will elaborate some major
ideas in spam detection and review the major data mining techniques
including supervised methods and unsupervised methods for spam
detection. We will also discuss the spam issue and the detection
techniques in sponsored search and adversarial information
retrieval. Lastly, we will analyze the challenges and opportunities
for data mining community.
Short Biography:
Jian Pei received his Ph.D. in Computing Science from Simon
Fraser University, Canada, in 2002. He is currently an Assistant
Professor of Computing Science at Simon Fraser University, Canada.
His research interests can be summarized as developing effective and
efficient data analysis techniques for novel data intensive
applications. Currently, he is interested in various techniques of
data mining, data warehousing, online analytical processing, and
database systems, as well as their applications in web search,
sensor networks, bioinformatics, privacy preservation, and
education. His current research has been supported in part by NSERC,
NSF, Microsoft, IBM, HP, CIBC, Michael Smith Foundation, and the SFU
Community Trust Endowment Fund. He has published prolifically in
refereed journals, conferences, and workshops. He is an associate
editor of IEEE Transactions on Knowledge and Data Engineering. He
has served regularly in the organization committees and the program
committees of many international conferences and workshops, and has
also been a reviewer for the leading academic journals in his
fields. He is senior members of the Association for Computing
Machinery (ACM) and the Institute of Electrical and Electronics
Engineers (IEEE). He is the recipient of the British Columbia
Innovation Council 2005 Young Innovator Award.
Bin Zhou is a Ph.D. student in School of Computing Science at Simon
Fraser University, Canada. His current research interests are in
database, data mining and information Retrieval, particularly in
data mining and privacy preserving techniques for social networks.
ZhaoHui Tang is a group program manager at Microsoft adCenter Labs,
where he manages a number of research projects related to paid
search and content advertisements. He is the main inventor of
Microsoft Keyword Services Platform -- the first web service
platform specialized on keyword technologies in the industry. Prior
to adCenter, he spent 6 years as a lead program manager in SQL
Server Business Intelligence group, mainly focusing on data mining
development. ZhaoHui Tang got his Ph.D. in France in 1996 on the
topic of database query processing. Prior joining Microsoft, he
worked in Sema Group based in Paris, leading an R\&D team doing data
mining project. He has numerous publications in both academic and
industrial journals such as VLDB and SQL Server Magazine. He is a
frequent speaker in database and business intelligence conferences
and often serves as committee members for those conferences. He
holds a guest professorship in Nan Kai University, China. He is a
co-author of the book "Data Mining with SQL Server 2005".
Dylan Hai Huang is a Program Manager in Microsoft adCenter Labs.
Currently, he is working on Microsoft Keyword Services Platform (KSP)
and its related applications. KSP packaged many advanced research
algorithms to a development platform and has been widely adopted in
search engine marketing and optimization community. He is pursuing
his Ph.D degree in Computing Science in Simon Fraser University.
Before joining adCenter Labs, he spent over 5 years in Microsoft
Business Intelligence groups, working on Microsoft Analysis Services
OLAP related technologies. He coauthored the book "MDX Solutions:
With Microsoft SQL Server Analysis Services 2005 and Hyperion
Essbase". His major research interests are data mining and machine
learning.
|
|
|