Related Conferences
4-6 April, 2013, Sydney, Australia
8-11 April, 2013, Brisbane, Australia
|
CountDown
Big Data School&Summit in Sydney
10.4.2013
-
23:59 o'clock
Countdown expired
Gold Sponsor
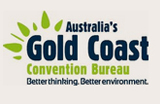
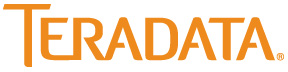
PAKDD2013
DETAILS OF KEYNOTE SPEAKERS
Dr Usama Fayyad

Chairman & CTO, Blue Kangaroo (ChoozOn Corp)
BigData and Predictive Analytics – Opportunities and Threats
Virtually all organizations are having to deal with Big Data in many contexts: marketing, operations, monitoring, performance, and even financial management. Big Data is characterized not just by its size, but by its Velocity and its Variety for which keeping up with the data flux, let alone its analysis, is challenging at best and impossible in many cases. In this talk I will cover some of the basics in terms of infrastructure and design considerations for effective an efficient BigData. In many organizations, the lack of consideration of effective infrastructure and data management leads to unnecessarily expensive systems that fail the cost/benefits analysis. We will refer to example frameworks and clarify the kinds of operations where Map-Reduce (Hadoop and and its derivatives) are appropriate and the situations where other infrastructure is needed to perform segmentation, prediction, analysis, and reporting appropriately – these being the fundamental operations in predictive analytics. We will then pay specific attention to on-line data and the unique challenges and opportunities represented there. We cover examples of Predictive Analytics over Big Data with case studies in eCommerce Marketing, on-line publishing and recommendation systems, and advertising targeting, and we conclude with some case studies in Social Network data. The main theme is that if the data mining and predictive analytics communities do not embrace BigData methods and systems, they will likely miss on huge opportunities to contribute scientifically and in an applied manner.
Usama M. Fayyad, Ph.D. is Chairman & CTO of ChoozOn Corporation/Blue Kangaroo -- a mobile search engine service that helps consumers find offers and deals through personalization and intelligent matching. In 2010 he was appointed by King Abdullah II of Jordan to lead the OASIS-500 as its Executive Chairman -- a tech startup investment fund that runs an accelerator/incubator, and angel investor network that aims to fund 500 Internet and Technology startups in the next 5 years. This effort is in collaboration with U.S. Department of State who also helps fund the entrepreneurship training program or Oasis500. In 2008, Fayyad founded Open Insights LLC, a data strategy, technology and consulting firm to help enterprises understand data strategy and deploy data-driven solutions that effectively and dramatically grow revenue and competitive advantages.
Up until September 2008, he was in Sunnyvale, CA as Yahoo!'s chief data officer & Executive VP responsible for Yahoo!'s global data strategy, architecting Yahoo!'s data policies and systems, prioritizing data investments, and managing the Company's data analytics and data processing infrastructure which processed over 25 Terabytes of data per day. Fayyad also founded and managed the Yahoo! Research Labs with offices around the world as the premier scientific research organization to develop the new sciences of the Internet, on-line marketing, and algorithmic Advertising. At Yahoo! he applied Big Data techniques to content and advertising targeting and built the world’s largest group of data scientist – helping Yahoo! grow its revenues for targeting by 20x in 4 years.
In 2003 Fayyad co-founded and led the DMX Group, a data mining and data strategy consulting and technology company that was acquired by Yahoo! in 2004. In early 2000, he co-founded and served as CEO of Audience Science (originally digiMine, Inc.), a data analysis and data mining company that is the leader in Behavioral Targeting and advertising networks. Fayyad's professional experience also includes five years spent leading the data mining and exploration group at Microsoft Research and building the data mining products for Microsoft's server division. From 1989 to 1996 Fayyad held a leadership role at NASA's Jet Propulsion Laboratory (JPL), where his work in the analysis and exploration of Big Data in scientific applications gathered from observatories, remote-sensing platforms and spacecraft garnered him the top research excellence award that Caltech awards to JPL scientists, as well as a U.S. Government medal from NASA.
Fayyad earned his Ph.D. in engineering from the University of Michigan, Ann Arbor (1991), and also holds BSE's in both electrical and computer engineering (1984); MSE in computer science and engineering (1986); and M.Sc. in mathematics (1989). He has published over 100 technical articles in the fields of data mining, Artificial Intelligence, machine learning, and databases. He holds over 30 patents, is a Fellow of the AAAI (Association for Advancement of Artificial Intelligence) and a Fellow of the ACM (Association of Computing Machinery), has edited two influential books on the data mining and launched and served as editor-in-chief of both the primary scientific journal in the field of data mining (Data Mining and Knowledge Discovery). He is ACM SIGKDD’s Chairman which runs the world’s premiere data science, big data, and data mining conferences: KDD, and was founding editor-in-chief of SIGKDD Explorations Newsletter.
Usama is an active angel investor in the U.S. and in the Middle East and specializes in early-stage tech companies. He is part of the U.S. Dept of State Delegation on Entrepreneurship in the Middle East and North Africa.
|
Professor Huan Liu

Computer Science and Engineering School of Computing, Informatics, and Decision Systems Engineering Ira A. Fulton Schools of Engineering, Arizona State University, USA
Some New Data-Mining Challenges with Social Media Data
People of all walks of life use social media for communications and networking. Their active participation in numerous and diverse online activities continually generates massive amounts of social media data. This undoubtedly “big” data presents new challenges to data mining. For illustrative purposes, we discuss two of them: (1) how to use linked social media data with the presence of varied relations for feature selection, and (2) how to ensure that patterns discovered from social media data are valid when no ground truth is available. We will introduce the intricacies of social media data, present original social-computing problems, deliberate approaches to mining social media data to gain insight from real-world applications and deepen our understanding, and exploit unique characteristics of social media data in developing novel algorithms and computational tools to advance research and development of social media mining.
Dr. Huan Liu is a professor of Computer Science and Engineering at Arizona State University. He obtained his Ph.D. in Computer Science at University of Southern California and B.Eng. in EECS at Shanghai JiaoTong University. He was recognized for excellence in teaching and research in Computer Science and Engineering at Arizona State University. His research interests are in data mining, machine learning, social computing, and artificial intelligence, investigating problems that arise in real-world applications with high-dimensional data of disparate forms. His well-cited publications include books, book chapters, encyclopedia entries as well as conference and journal papers. He serves on journal editorial/advisory boards and numerous conference program committees. He is a Fellow of IEEE and a member of several professional societies.
|
Professor Qiang Yang

Huawei Noah's Ark Lab Science and Technology Park, Shatin, Hong Kong http://www.noahlab.com.hk
Big Data, Lifelong Machine Learning and Transfer Learning
A major challenge in today's world is the Big Data problem, which manifests itself in Web and Mobile domains as rapidly changing and heterogeneous data streams. A data-mining system must be able to cope with the influx of changing data in a continual manner. This calls for Lifelong Machine Learning, which in contrast to the traditional one-shot learning, should be able to identify the learning tasks at hand and adapt to the learning problems in a sustainable manner. A foundation for lifelong machine learning is transfer learning, whereby knowledge gained in a related but different domain may be transferred to benefit learning for a current task. To make effective transfer learning, it is important to maintain a continual and sustainable channel in the life time of a user in which the data are annotated. In this talk, I outline the lifelong machine learning situations, give several examples of transfer learning and applications for lifelong machine learning, and discuss cases of successful extraction of data annotations to meet the Big Data challenge.
Qiang Yang is the head of Huawei Noah's Ark Research Lab and a professor in the Department of Computer Science and Engineering, Hong Kong University of Science and Technology. His research interests are data mining and artificial intelligence including machine learning, planning and activity recognition. He is a fellow of IEEE, IAPR and AAAS. He received his PhD from Computer Science Department of the University of Maryland, College Park in 1989. He had been an assistant/associate professor at the University of Waterloo between 1989 and 1995, and a professor and NSERC Industrial Research Chair at Simon Fraser University in Canada from 1995 to 2001. He was an invited speaker at IJCAI 2009, ACL 2009, ACML 2009 and ADMA 2008 and 2012, SDM 2012, WSDM 2013, etc. He was elected as a vice chair of ACM SIGART in July 2010. He is the founding Editor in Chief of the ACM Transactions on Intelligent Systems and Technology (ACM TIST), and is on the editorial board of IEEE Intelligent Systems and several other international journals. He has served as a PC co-chair and general co-chair of several international conferences, including ACM KDD 2010 and 2012, ACM RecSys 2013, ACM IUI 2010, etc. He serves as an IJCAI trustee and will be the PC chair for IJCAI 2015.
|
|
DETAILS OF INVITED SPEAKERS
Dr. Alexandros Batsakis
Teradata

Big Data Analytics Infrastructure and Applications for the Enterprise
This invited talk focuses on working solutions for big data infrastructure and analytics as well as real world case studies. The convergence of analytic logic with databases imposes severe performance and usability limitations for big data analytic processing. However, the advent of a massively parallel shared-nothing analytic database helps overcome the performance, development and analytic limitations associated with existing hardware and software implementations for big data.
This talk discusses a big data infrastructure, system architecture and introduces the SQL/MapReduce (SQL/MR) framework. Furthermore, the SQL/MR model of computation facilitates highly scalable computations within the database for big data analytics. Therefore, a key aspect of this talk intends to cover a diversity of real-world use cases highlighting digital marketing optimization, social network analysis, fraud detection, and machine data analysis.
Dr. Alexandros Batsakis is a Big Data Engineer and senior member of the engineering team at Teradata-Aster. His work focuses on management, performance and fault-tolerance of big data sets. Prior to joining Aster, Alexandros was a key developer of a next-generation parallel file system (pNFS), implementing parts of both the client and the server in the Linux kernel at NetApp Inc.
Alexandros holds a PhD in Computer Science from the Department of Computer Science at Johns Hopkins University working at the premier academic research lab for storage systems. His actual research improves the performance on network file systems through scheduling memory optimizations and read/write cooperative caching. This work on adaptive performance management for network data protocols culminated in a Congestion-Aware Network File System. He earned his Bachelors degree from the Department of Informatics and Telecommunications at University of Athens working on the semantic description and discovery of web services.
|
|
|